Early Challenges of Utilising MCP Servers
Author
Leo Messen
Date Published
Categories

Model Context Protocol (MCP) is a recently introduced open standard designed to facilitate seamless interactions between AI models and external tools and resources. Recently, this protocol has been generating a lot of attention due to its promise of empowering AI models with tools on a scale that wasn't really possible before. By providing a standard way that AI models can interact with tools and resources, developers can utilise MCP servers developed by someone else rather than writing their own tools. This saves developers time and allows them to utilise a wealth of tools developed by other developers, allowing them to build more powerful AI applications.
However, despite all the promise that this protocol shows, at this point, there are some limitations that impede the full utilisation of MCP servers amongst developers. This stems from the fact that, until recently, MCP servers were meant to run locally on your machine to aid locally running MCP clients. But, after its recent surge in popularity, developers are looking to utilise MCP servers in their current applications and are facing some significant challenges and limitations.
Current Challenges and Limitations
- Single-Tenant and Stateful Design: Most MCP servers are designed for single-tenant use and maintain stateful interactions. This architecture is not suited for scaling applications or accessing MCP servers from multiple clients.
- Deployment Constraints: Deploying MCP servers in serverless environments is particularly challenging under the current design. The easiest way to stand up MCP servers currently is utilising long-running containers communicating over SSE. While manageable with a limited number of MCP servers, this approach quickly becomes unreasonable when you want to offer a larger amount of MCP servers for your language models.
- Authentication and Authorisation Concerns: Although the recent integration of OAuth 2.0 into MCP represents a step in the right direction, almost all MCP servers lack support for passing authentication data inline, leading to insecure storage of authentication information on servers. This also means that each running MCP server is tied to one set of credentials which is insecure because access to the server automatically grants access to the credentials. It's also not very useful for multi-agent or multi-user systems where many different people/agents need to use the same tools, across different accounts, at the same time.
The Model Context Protocol represents a significant step toward standardising interactions between AI models and external systems. Despite some of the limitations we have explored in this blog, it still remains a promising new technology with lots of potential to empower AI applications. However, to fully realise its potential, addressing existing challenges related to deployment, security, and scalability is essential. Continued research, development, and community engagement will be crucial in refining MCP to meet the evolving needs of AI applications.
As we continue to follow the development of MCP, stay tuned for future blog posts on how to securely and effectively utilise MCP in enterprise environments.
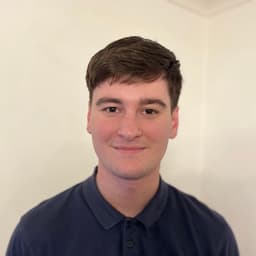